Documents
Poster
NOVEL METRIC LEARNING FOR NON-PARALLEL VOICE CONVERSION
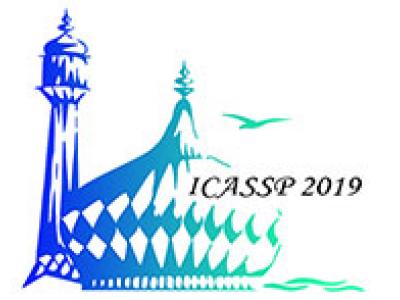
- Citation Author(s):
- Submitted by:
- Nirmesh Shah
- Last updated:
- 8 May 2019 - 8:07am
- Document Type:
- Poster
- Document Year:
- 2019
- Event:
- Presenters:
- Nirmesh Shah
- Paper Code:
- MLSP-P15.2
- Categories:
- Log in to post comments
Obtaining aligned spectral pairs in case of non-parallel data for stand-alone Voice Conversion (VC) technique is a challenging research problem. Unsupervised alignment algorithm, namely, an Iterative combination of a Nearest Neighbor search step and a Conversion step Alignment (INCA) iteratively tries to align the spectral features by minimizing the Euclidean distance metric between the intermediate converted and the target spectral feature vectors. However, the Euclidean distance may not correlate well with the perceptual distance between the two (sound or visual) patterns in a given feature space. In this paper, we propose to learn distance metric using Large Margin Nearest Neighbor (LMNN) technique that gives a minimum distance for the same phoneme uttered by the different speakers and more distance for the different set of phonemes. This learned metric is then used for finding the NN pairs in the INCA. Furthermore, we propose to use this learned metric only for the first iteration in the INCA, since the intermediate converted features (which are not the actual acoustic features) may not behave well w.r.t. the learned metric. We obtained on an average 7.93 % relative improvement in Phonetic Accuracy (PA). This is reflected positively in subjective and objective evaluations.