Documents
Poster
One TTS Alignment To Rule Them All
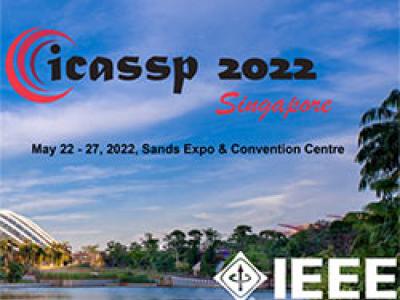
- Citation Author(s):
- Submitted by:
- Rohan Badlani
- Last updated:
- 15 May 2022 - 7:03pm
- Document Type:
- Poster
- Document Year:
- 2022
- Event:
- Presenters:
- Rohan Badlani
- Paper Code:
- SPE-3.6:
- Categories:
- Log in to post comments
Speech-to-text alignment is a critical component of neural text-to-speech (TTS) models. Autoregressive TTS models typically use an attention mechanism to learn these alignments on-line. However, these alignments tend to be brittle and often fail to generalize to long utterances and out-of-domain text, leading to missing or repeating words. Most non-autoregressive end-to-end TTS models rely on durations extracted from external sources. In this paper we leverage the alignment mechanism proposed in RAD-TTS and demonstrate its applicability to wide variety of neural TTS models. The alignment learning framework combines the forward-sum algorithm, Viterbi algorithm, and an efficient static prior. In our experiments, the framework improves all tested TTS architectures, both autoregressive (Flowtron, Tacotron 2) and non-autoregressive (FastPitch, FastSpeech 2, RAD-TTS). Specifically, it improves alignment convergence speed, simplifies the training pipeline by eliminating need for external aligners, enhances robustness to errors on long utterances and improves the perceived speech synthesis quality, as judged by human evaluators.