Documents
Poster
OPENFEAT: Improving Speaker Identification by Open-set Few-shot Embedding Adaptation with Transformer
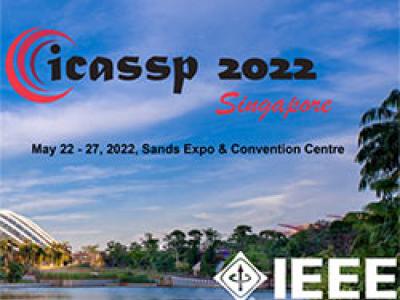
- Citation Author(s):
- Submitted by:
- Kishan KC
- Last updated:
- 9 May 2022 - 11:36am
- Document Type:
- Poster
- Document Year:
- 2022
- Event:
- Presenters:
- Kishan K C
- Paper Code:
- SPE-37.2
- Categories:
- Keywords:
- Log in to post comments
Household speaker identification with few enrollment utterances is an important yet challenging problem, especially when household members share similar voice characteristics and room acoustics. A common embedding space learned from a large number of speakers is not universally applicable for the optimal identification of every speaker in a household. In this work, we first formulate household speaker identification as a few-shot open-set recognition task and then propose a novel embedding adaptation framework to adapt speaker representations from the given universal embedding space to a household-specific embedding space using a set-to-set function, yielding better household speaker identification performance. With our algorithm, Open-set Few-shot Embedding Adaptation with Transformer (openFEAT), we observe that the speaker identification equal error rate (IEER) on simulated households with 2 to 7 hard-to-discriminate speakers is reduced by 23% to 31% relative.