Documents
Poster
Out-of-label Suppression Dictionary Learning with Cluster Regularization
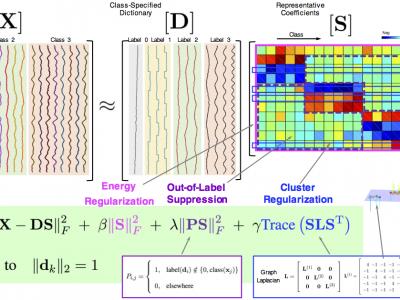
- Citation Author(s):
- Submitted by:
- Yuantao Gu
- Last updated:
- 6 December 2016 - 11:06am
- Document Type:
- Poster
- Document Year:
- 2016
- Event:
- Presenters:
- Yuantao Gu
- Paper Code:
- CSDL-P3.4
- Categories:
- Log in to post comments
This paper addresses the problem of learning a discriminative dictionary from training signals. Given a structured dictionary, each atom of which has its corresponding label, one signal should be mainly constructed by its closely associated atoms. Besides the representations for the same class ought to be very close to form a cluster. Thus we present out-of-label suppression dictionary model with cluster regularization to amplify the discriminative power. Upon out-of-label suppression, we don't adopt $l_0$-norm or $l_1$-norm for regularization. Meanwhile, two simple classifier are developed to take full advantage of the learnt dictionary. The effectiveness of the proposed dictionary model has been evaluated on two popular visual benchmarks.
GlobalSIP 2016.pdf
