Documents
Presentation Slides
OUTLIER REMOVAL FOR ENHANCING KERNEL-BASED CLASSIFIER VIA THE DISCRIMINANT INFORMATION
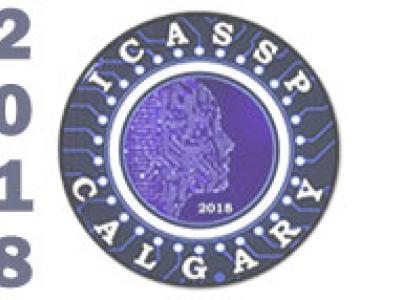
- Citation Author(s):
- Submitted by:
- Mert Al
- Last updated:
- 14 April 2018 - 9:10pm
- Document Type:
- Presentation Slides
- Document Year:
- 2018
- Event:
- Presenters:
- Mert Al
- Paper Code:
- MLSP-L4.2
- Categories:
- Log in to post comments
Pattern recognition on big data can be challenging for kernel machines as the complexity grows with the squared number of training samples. In this work, we overcome this hurdle via the outlying data sample removal pre-processing step. This approach removes less informative data samples and trains the kernel machines only with the remaining data, and hence, directly reduces the complexity by reducing the number of training samples. To enhance the classification performance, the outlier removal process is done such that the discriminant information of the data is mostly intact. This is achieved via the novel Outlier-Removal Discriminant Information (ORDI) metric, which measures the contribution of each sample toward the discriminant information of the dataset. Hence, the ORDI metric can be used together with the simple filter method to effectively remove insignificant outliers to both reduce the computational cost and enhance the classification performance. We experimentally show on two real-world datasets at the sample removal ratio of 0.2 that, with outlier removal via ORDI, we can simultaneously (1) improve the accuracy of the classifier by 1%, and (2) provide significant saving on the total running time by 1.5x and 2x on the two datasets. Hence, ORDI can provide a win-win situation in this performance-complexity tradeoff of the kernel machines for big data analysis.