Documents
Presentation Slides
Pairwise Adjacency Matrix on Spatial Temporal Graph Convolution Network for Skeleton-based Two-Person Interaction Recognition
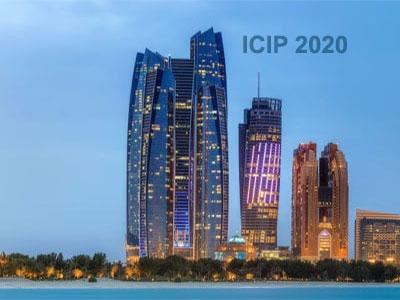
- Citation Author(s):
- Submitted by:
- Chao-Lung Yang
- Last updated:
- 4 November 2020 - 5:41am
- Document Type:
- Presentation Slides
- Document Year:
- 2020
- Event:
- Presenters:
- Chao-Lung Yang
- Categories:
- Log in to post comments
Spatial-temporal graph convolutional networks (ST-GCN) have achieved outstanding performances on human action recognition, however, it might be less superior on a two-person interaction recognition (TPIR) task due to the relationship of each skeleton is not considered. In this study, we present an improvement of the ST-GCN model that focused on TPIR by employing the pairwise adjacency matrix to capture the relationship of person-person skeletons (ST-GCN-PAM). To validate the effectiveness of the proposed ST-GCN-PAM model on TPIR, experiments were conducted on NTU RGB+D 120. Additionally, the model was also examined on the Kinetics dataset and NTU RGB+D 60. The results show that the proposed ST-GCN-PAM outperforms the-state-of-the-art methods on mutual action of NTU RGB+D 120 by achieving 83.28% (cross-subject) and 88.31% (cross-view) accuracy. The model is also superior to the original ST-GCN on the multi-human action of the Kinetics dataset by achieving 41.68% in Top-1 and 88.91% in Top-5.