Documents
Presentation Slides
Pairwise Feature Learning for Unseen Plant Disease Recognition
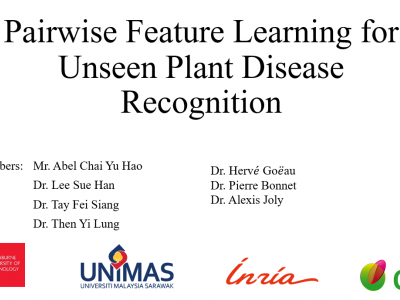
- DOI:
- 10.60864/ts6p-0a26
- Citation Author(s):
- Submitted by:
- Abel Yu Hao Chai
- Last updated:
- 17 November 2023 - 12:05pm
- Document Type:
- Presentation Slides
- Document Year:
- 2023
- Event:
- Presenters:
- Abel Chai Yu Hao
- Paper Code:
- 1274
- Categories:
- Keywords:
- Log in to post comments
With the advent of Deep Learning, people have begun to use it with computer vision approaches to identify plant diseases on a large scale targeting multiple crops and diseases. However, this requires a large amount of plant disease data, which is often not readily available, and the cost of acquiring disease images is high. Thus, developing a generalized model for recognizing unseen classes is very important and remains a major challenge to date. Existing methods solve the problem with general supervised recognition tasks based on the seen composition of the crop and the disease. However, ignoring the composition of unseen classes during model training can lead to a reduction in model generalisation. Therefore, in this work, we propose a new approach that leverages the visual features of crop and disease from the seen composition, using them to learn the features of unseen crop-disease composition classes. We show that our proposed method can improve the classification performance of these unseen classes and outperform the state-of-the-art in the identification of multiple crop-diseases.