Documents
Presentation Slides
PARALLEL WAVEGAN: A FAST WAVEFORM GENERATION MODEL BASED ON GENERATIVE ADVERSARIAL NETWORKS WITH MULTI-RESOLUTION SPECTROGRAM
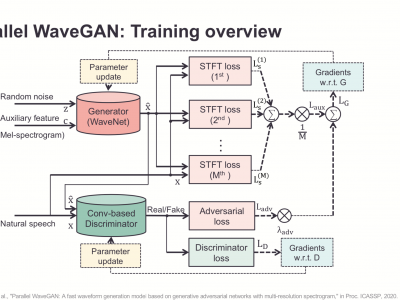
- Citation Author(s):
- Submitted by:
- Ryuichi Yamamoto
- Last updated:
- 13 May 2020 - 10:56pm
- Document Type:
- Presentation Slides
- Document Year:
- 2020
- Event:
- Presenters:
- Ryuichi Yamamoto
- Paper Code:
- SPE-L5.5
- Categories:
- Log in to post comments
We propose Parallel WaveGAN, a distillation-free, fast, and small-footprint waveform generation method using a generative adversarial network. In the proposed method, a non-autoregressive WaveNet is trained by jointly optimizing multi-resolution spectrogram and adversarial loss functions, which can effectively capture the time-frequency distribution of the realistic speech waveform. As our method does not require density distillation used in the conventional teacher-student framework, the entire model can be easily trained. Furthermore, our model is able to generate high-fidelity speech even with its compact architecture. In particular, the proposed Parallel WaveGAN has only 1.44 M parameters and can generate 24 kHz speech waveform 28.68 times faster than real-time on a single GPU environment. Perceptual listening test results verify that our proposed method achieves 4.16 mean opinion score within a Transformer-based text-to-speech framework, which is comparative to the best distillation-based Parallel WaveNet system.