Documents
Presentation Slides
PARAMETER-EFFICIENT ADAPTATION FOR COMPUTATIONAL IMAGING
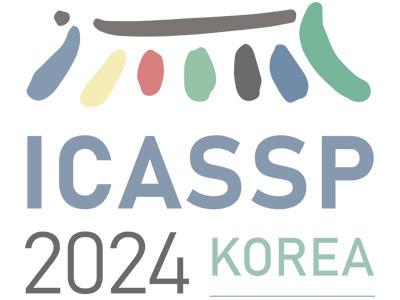
- DOI:
- 10.60864/hk0h-t525
- Citation Author(s):
- Submitted by:
- Nebiyou Yismaw
- Last updated:
- 6 June 2024 - 10:28am
- Document Type:
- Presentation Slides
- Document Year:
- 2024
- Event:
- Presenters:
- Nebiyou Yismaw
- Paper Code:
- 4263
- Categories:
- Log in to post comments
Deep learning-based methods provide remarkable performance in a number of computational imaging problems. Examples include end-to-end trained networks that map measurements to unknown signals, plug-and-play (PnP) methods that use pretrained denoisers as image prior, and model-based unrolled networks that train artifact removal blocks. Many of these methods lack robustness and fail to generalize with distribution shifts in data, measurements, and noise. In this paper, we present a simple framework to perform domain adaptation as data and measurement distribution shifts. Our method learns a small number of factors to add in a pretrained model to bridge the gap in performance. We present a number of experiments on accelerated magnetic resonance imaging (MRI) reconstruction and image deblurring to demonstrate that our method requires a small amount of memory and parameter overhead to adapt to new domains.