Documents
Presentation Slides
Patient-Specific Modeling of Daily Activity Patterns for Unsupervised Detection of Psychotic and Non-Psychotic Relapses
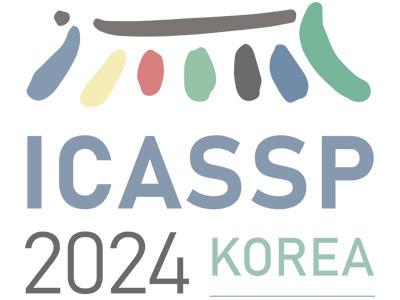
- DOI:
- 10.60864/4466-k391
- Citation Author(s):
- Submitted by:
- Sven Gronauer
- Last updated:
- 6 June 2024 - 10:27am
- Document Type:
- Presentation Slides
- Document Year:
- 2024
- Event:
- Presenters:
- Sven Gronauer
- Paper Code:
- GC-L2.2
- Categories:
- Log in to post comments
In this paper, we present our submission to the 2nd e-Prevention Grand Challenge hosted at ICASSP 2024. The objective posed in the challenge was to identify psychotic and non- psychotic relapses in patients using biosignals captured by wearable sensors. Our proposed solution is an unsupervised anomaly detection approach based on Transformers. We train individual models for each patient to predict the timestamps of biosignal measurements on non-relapse days, implicitly modeling normal daily routines. The models’ mean-normalized prediction errors are then used as indica- tors of atypical behavior and, thus, risk of relapse. Our final submission ranked 3rd on detecting non-psychotic relapses (Track 1) and 1st on detecting psychotic relapses (Track 2).