Documents
Presentation Slides
POINT OF CARE IMAGE ANALYSIS FOR COVID-19
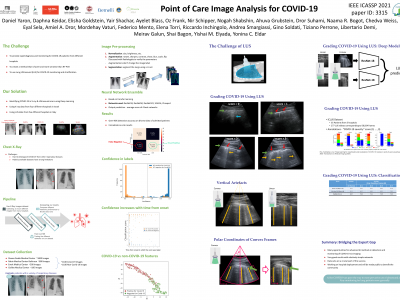
- Citation Author(s):
- Submitted by:
- Shai Bagon
- Last updated:
- 22 June 2021 - 2:37am
- Document Type:
- Presentation Slides
- Document Year:
- 2021
- Event:
- Presenters:
- Daniel Yaron
- Paper Code:
- SS-4.2
- Categories:
- Log in to post comments
Early detection of COVID-19 is key in containing the pandemic. Disease detection and evaluation based on imaging is fast and cheap and therefore plays an important role in COVID-19 handling. COVID-19 is easier to detect in chest CT, however, it is expensive, non-portable, and difficult to disinfect, making it unfit as a point-of-care (POC) modality. On the other hand, chest X-ray (CXR) and lung ultrasound (LUS) are widely used, yet, COVID-19 findings in these modalities are not always very clear. Here we train deep neural networks to significantly enhance the capability to detect, grade and monitor COVID-19 patients using CXRs and LUS. Collaborating with several hospitals in Israel we collect a large dataset of CXRs and use this dataset to train a neural network obtaining above 90% detection rate for COVID-19. In addition, in collaboration with ULTRa (Ultrasound Laboratory Trento, Italy) and hospitals in Italy we obtained POC Ultrasound data with annotations of the severity of disease and trained a deep network for automatic severity grading.