Documents
Poster
Point Cloud Upsampling via Dynamic Graph Scattering Transform
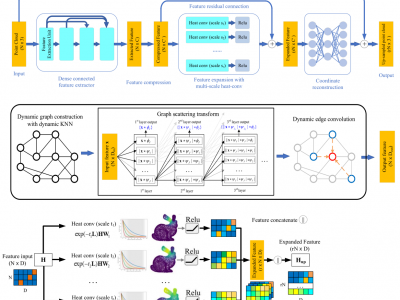
- DOI:
- 10.60864/0b5b-y843
- Citation Author(s):
- Submitted by:
- Shiyu Liu
- Last updated:
- 17 November 2023 - 12:05pm
- Document Type:
- Poster
- Document Year:
- 2023
- Event:
- Presenters:
- Liu Shiyu
- Paper Code:
- 3144
- Categories:
- Log in to post comments
Point cloud upsampling is a challenging task to generate dense and uniform point clouds from sparse and irregular inputs. Existing deep learning based methods usually leverage graph convolution networks (GCNs) to learn features for expansion and reconstruction but suffer from the over-smoothing issue by discarding the high-frequency components. In this paper, we design a novel dynamic graph scattering transform (GST) to achieve multi-scale feature extraction for point cloud upsampling. The dynamic GST can accommodate to the K-nearest neighbor (KNN)-based feature graph dynamically constructed for point clouds to achieve multi-resolution band-pass representations. Consequently, a feature extractor with dense connection is developed based on the dynamic GST such that low-frequency and high-frequency components can be sufficiently combined for subsequent feature expansion and reconstruction. To our best knowledge, this paper is the first to introduce graph scattering transform for representation learning with dynamic feature graphs in point cloud upsampling. Experimental results on the PU1K dataset show that the proposed network based on dynamic GST achieve promising performance under various metrics especially for sparse point clouds.
poster.pptx
