Documents
Presentation Slides
Presentation Slides
Polar Feature Based Deep Architectures for Automatic Modulation Classification Considering Channel Fading
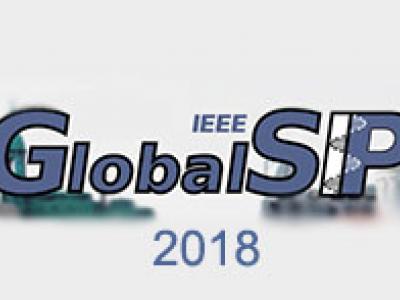
- Citation Author(s):
- Submitted by:
- Chieh-Fang Teng
- Last updated:
- 27 November 2018 - 6:59am
- Document Type:
- Presentation Slides
- Document Year:
- 2018
- Event:
- Presenters:
- Chieh-Fang Teng
- Paper Code:
- DLW-L.1.1
- Categories:
- Log in to post comments
To develop intelligent receivers, automatic modulation classification (AMC) plays an important role for better spectrum utilization. The emerging deep learning (DL) technique has received much attention in AMC due to its superior performance in classifying data with deep structure. In this work, a novel polar-based deep learning architecture with channel compensation network (CCN) is proposed. Our test results show that learning features from polar domain (r-θ) can improve recognition accuracy by 5% and reduce training overhead by 48%. Besides, the proposed CCN is also robust to channel fading, such as amplitude and phase offsets, and can improve the recognition accuracy by 14% under practical channel environments.