Documents
Poster
poster
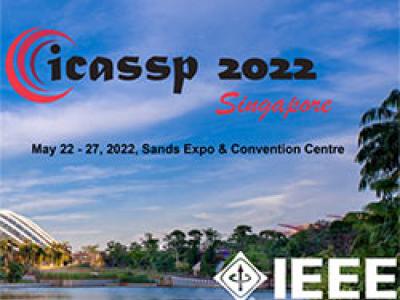
- Citation Author(s):
- Submitted by:
- Baoshun Shi
- Last updated:
- 4 May 2022 - 9:34pm
- Document Type:
- Poster
- Document Year:
- 2022
- Event:
- Presenters:
- Baoshun Shi
- Paper Code:
- ICASSP-5618
- Categories:
- Log in to post comments
Recently, the PnP-GAP algorithm has achieved remarkable reconstruction quality for snapshot compressive imaging (SCI), and its convergence has been proven based on the condition of diminishing noise levels and the assumption of
bounded denoisers. However, most of deep denoisers are difficult to be proven as bounded denoisers due to the lack of interpretability of deep network architectures. To address this issue, a trainable bounded denoiser using double tight frame network for SCI is proposed. Firstly, to achieve higher denoising ability, we extend the single-layer tight frame to the two-layer one dubbed as double tight frame. Then, we elaborate a deep shrinkage network (DSN) for improving the generalization ability of the plugged deep denoiser to noise levels. Finally, we employ the double tight frame equipped with DSN to construct a Gaussian denoiser that can be trained in a supervised learning manner. We prove this trainable denoiser is bounded theoretically, and demonstrate that the PnP-GAP framework plugged by this denoiser can achieve competitive reconstruction quality compared with benchmark SCI algorithms empirically.