Documents
Poster
POSTER - Grad-CAM-Inspired Interpretation of Nearfield Acoustic Holography using Physics-Informed Explainable Neural Network
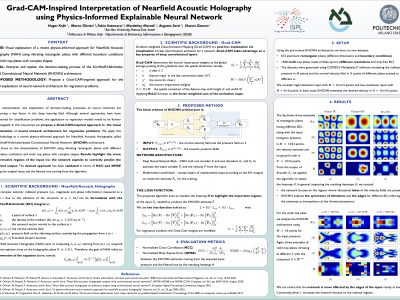
- DOI:
- 10.60864/xrr1-f698
- Citation Author(s):
- Submitted by:
- Marco Olivieri
- Last updated:
- 17 November 2023 - 12:07pm
- Document Type:
- Poster
- Document Year:
- 2023
- Event:
- Presenters:
- Marco Olivieri
- Paper Code:
- 3898 (AASP-P6.12)
- Categories:
- Log in to post comments
The interpretation and explanation of decision-making processes of neural networks are becoming a key factor in the deep learning field. Although several approaches have been presented for classification problems, the application to regression models needs to be further investigated. In this manuscript we propose a Grad-CAM-inspired approach for the visual explanation of neural network architecture for regression problems. We apply this methodology to a recent physics-informed approach for Nearfield Acoustic Holography, called Kirchhoff-Helmholtz-based Convolutional Neural Network (KHCNN) architecture. We focus on the interpretation of KHCNN using vibrating rectangular plates with different boundary conditions and violin top plates with complex shapes. Results highlight the more informative regions of the input that the network exploits to correctly predict the desired output. The devised approach has been validated in terms of NCC and NMSE using the original input and the filtered one coming from the algorithm.