Documents
Poster
Poster for the paper "Revisiting Deep Generalized Canonical Correlation Analysis"
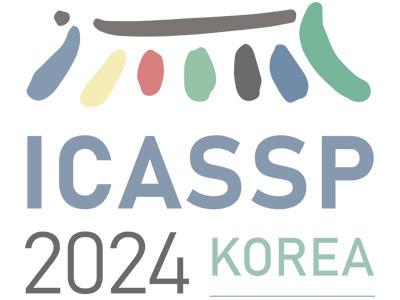
- DOI:
- 10.60864/0fak-tp63
- Citation Author(s):
- Submitted by:
- Paris Karakasis
- Last updated:
- 6 June 2024 - 10:54am
- Document Type:
- Poster
- Document Year:
- 2024
- Event:
- Presenters:
- Paris A. Karakasis
- Paper Code:
- MLSP-L21.5
- Categories:
- Log in to post comments
Canonical correlation analysis (CCA) is a classic statistical method for discovering latent co-variation that underpins two or more observed random vectors. Several extensions and variations of CCA have been proposed that have strengthened our capabilities in terms of revealing common random factors from multiview datasets. In this work, we first revisit the most recent deterministic extensions of deep CCA and highlight the strengths and limitations of these state-of-the-art methods. Some methods allow trivial solutions, while others can miss weak common factors. Others overload the problem by also seeking to reveal what is not common among the views -- i.e., the private components that are needed to fully reconstruct each view. The latter tends to overload the problem and its computational and sample complexities. Aiming to improve upon these limitations, we design a novel and efficient formulation that alleviates some of the current restrictions. The main idea is to model the private components as conditionally independent given the common ones, which enables the proposed compact formulation. In addition, we also provide a sufficient condition for identifying the common random factors. Judicious experiments with synthetic and real datasets showcase the validity of our claims and the effectiveness of the proposed approach.