Documents
Poster
[Poster] A Variable Smoothing for Nonconvexly Constrained Nonsmooth Optimization with Application to Sparse Spectral Clustering
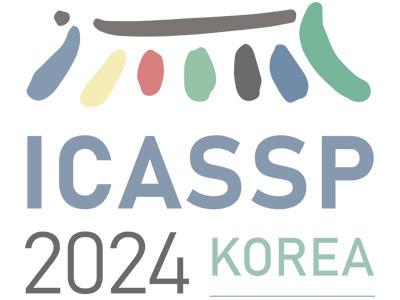
- DOI:
- 10.60864/vwwj-ah57
- Citation Author(s):
- Submitted by:
- Keita Kume
- Last updated:
- 4 November 2024 - 11:55pm
- Document Type:
- Poster
- Document Year:
- 2024
- Event:
- Presenters:
- Keita Kume
- Paper Code:
- SPTM-P9.10
- Categories:
- Log in to post comments
We propose a variable smoothing algorithm for solving nonconvexly constrained nonsmooth optimization problems. The target problem has two issues that need to be addressed: (i) the nonconvex constraint and (ii) the nonsmooth term. To handle the nonconvex constraint, we translate the target problem into an unconstrained problem by parameterizing the nonconvex constraint in terms of a Euclidean space. We show that under a certain condition, these problems are equivalent in view of finding a stationary point. To find a stationary point of the parameterized problem, the proposed algorithm performs the gradient descent update for the smoothed version of the parameterized problem with replacement of the nonsmooth function by the Moreau envelope, inspired by a variable smoothing algorithm [Böhm-Wright, J. Optim. Theory Appl., 2021] specialized for unconstrained nonsmooth optimization. We also present a convergence analysis of the proposed algorithm as well as its application to a nonconvex reformulation of the sparse spectral clustering.