Documents
Poster
Poster for Zero-Shot Learning of Intent Embeddings for Expansion by Convolutional Deep Structured Semantic Models
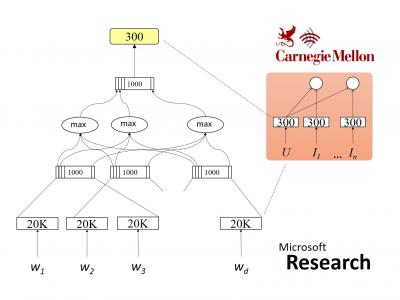
- Citation Author(s):
- Submitted by:
- Yun-Nung Chen
- Last updated:
- 31 March 2016 - 7:30pm
- Document Type:
- Poster
- Document Year:
- 2016
- Event:
- Presenters:
- Yun-Nung Chen
- Paper Code:
- HLT-P1.1
- Categories:
- Log in to post comments
The recent surge of intelligent personal assistants motivates spoken language understanding of dialogue systems. However, the domain constraint along with the inflexible intent schema remains a big issue. This paper focuses on the task of intent expansion, which helps remove the domain limit and make an intent schema flexible. A convolutional deep structured semantic model (CDSSM) is applied to jointly learn the representations for human intents and associated utterances. Then it can flexibly generate new intent embeddings without the need of training samples and model-retraining, which bridges the semantic relation between seen and unseen intents and further performs more robust results. Experiments show that CDSSM is capable of performing zero-shot learning effectively, e.g. generating embeddings of previously unseen intents, and therefore expand to new intents without re-training, and outperforms other semantic embeddings. The discussion and analysis of experiments provide a future direction for reducing human effort about annotating data and removing the domain constraint in spoken dialogue systems.