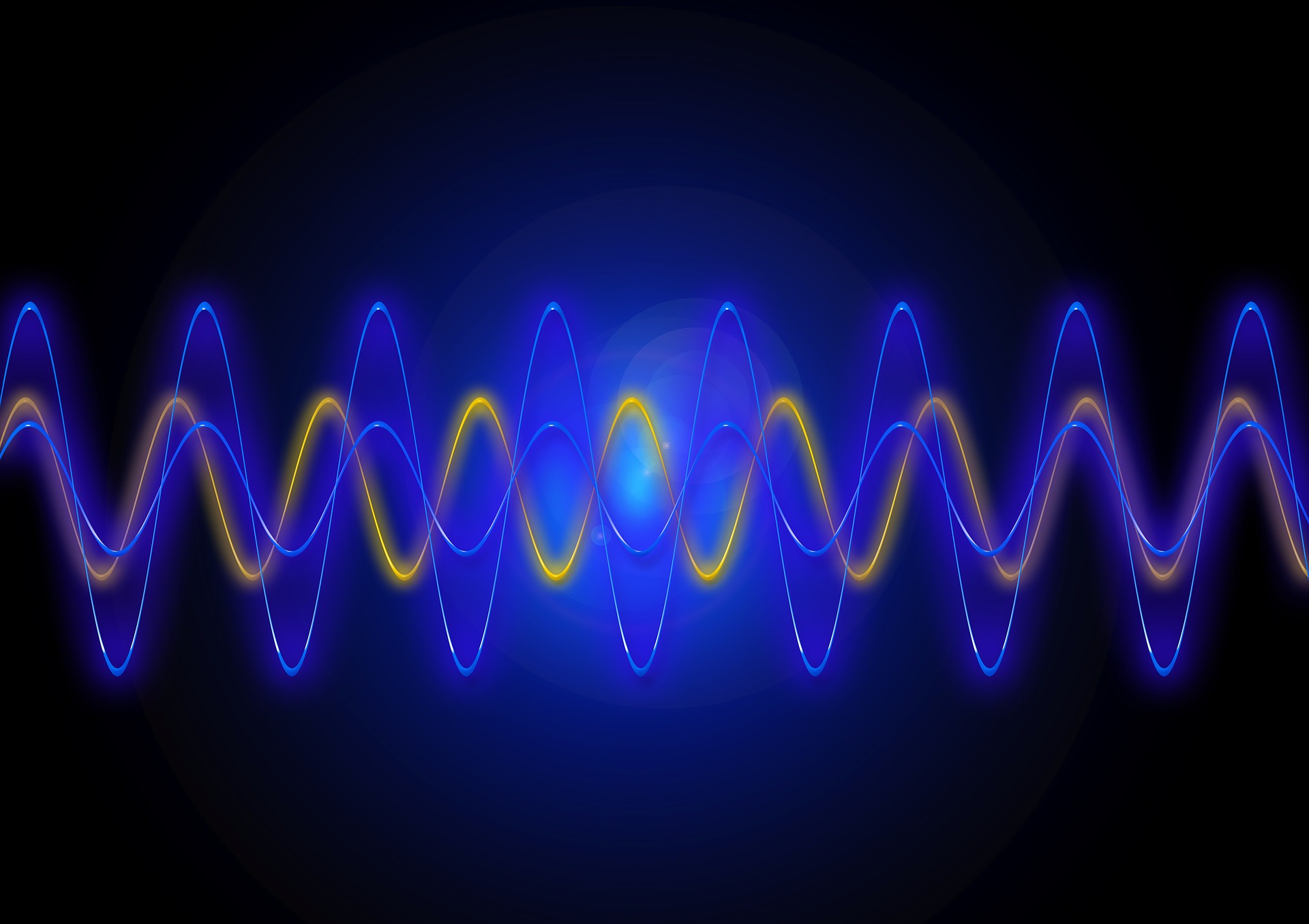
- Read more about Seeing Through the Conversation: Audio-visual Speech Separation based on Diffusion Model
- Log in to post comments
The objective of this work is to extract the target speaker’s voice from a mixture of voices using visual cues. Existing works on audio-visual speech separation have demonstrated their performance with promising intelligibility, but maintaining naturalness remains challenging. To address this issue, we propose AVDiffuSS, an audio-visual speech separation model based on a diffusion mechanism known for its capability to generate natural samples. We also propose a cross-attention-based feature fusion mechanism for an effective fusion of the two modalities for diffusion.
- Categories:
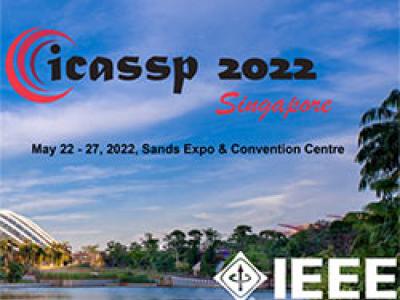
- Read more about Multimodal Transformer With Learnable Frontend and Self Attention for Emotion Recognition
- Log in to post comments
In this work, we propose a novel approach for multi-modal emotion recognition from conversations using speech and text. The audio representations are learned jointly with a learnable audio front-end (LEAF) model feeding to a CNN based classifier. The text representations are derived from pre-trained bidirectional encoder representations from transformer (BERT) along with a gated recurrent network (GRU). Both the textual and audio representations are separately processed using a bidirectional GRU network with self-attention.
- Categories:
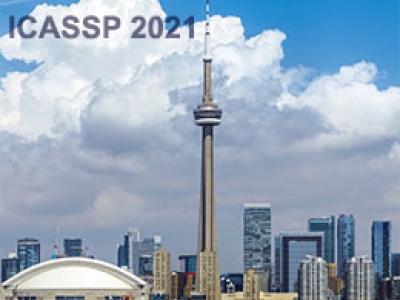
- Read more about LEARNING TO SELECT CONTEXT IN A HIERARCHICAL AND GLOBAL PERSPECTIVE FOR OPEN-DOMAIN DIALOGUE GENERATION
- 1 comment
- Log in to post comments
- Categories:
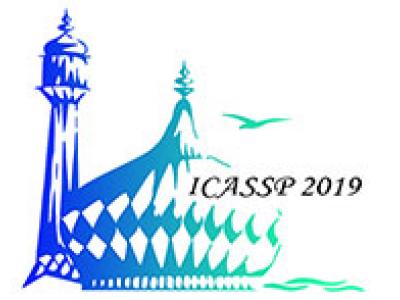
- Read more about Joint On-line Learning of a Zero-Shot Spoken Semantic Parser and a Reinforcement Learning Dialogue Manager
- Log in to post comments
Despite many recent advances for the design of dialogue systems, a true bottleneck remains the acquisition of data required to train its components. Unlike many other language processing applications, dialogue systems require interactions with users, therefore it is complex to develop them with pre-recorded data. Building on previous works, on-line learning is pursued here as a most convenient way to address the issue. Data collection, annotation and use in learning algorithms are performed in a single process.
- Categories:
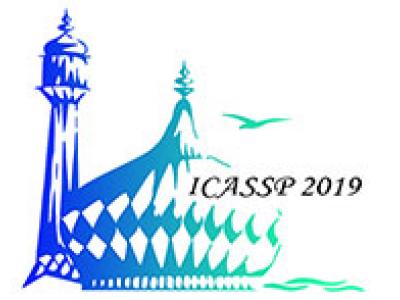
- Read more about Improving Human-Computer Interaction in Low-Resource Settings with Text-to-Phonetic Data Augmentation
- Log in to post comments
Off-the-shelf speech recognizers are error-prone in specialized domains; we aim to mitigate the impact of these errors for downstream classification tasks without in-domain speech training data, by augmenting available typewritten text training data with inferred phonetic information. We apply our method to mitigate the effects of the lack of speech training data when converting a typed chatbot to a spoken language interface.
Paper available here: https://ieeexplore.ieee.org/document/8682550
- Categories:
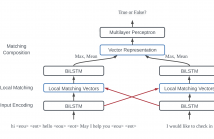
- Read more about SEQUENTIAL MATCHING MODEL FOR END-TO-END MULTI-TURN RESPONSE SELECTION
- Log in to post comments
- Categories:
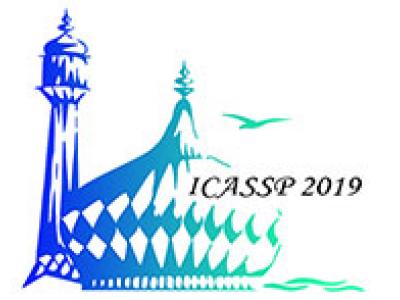
- Read more about Poster of 'DEEP HYBRID NETWORKS BASED RESPONSE SELECTION FOR MULTI-TURN DIALOGUE SYSTEMS'
- Log in to post comments
- Categories:
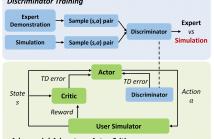
- Read more about Adversarial Advantage Actor-Critic Model for Task-Completion Dialogue Policy Learning
- Log in to post comments
This paper presents a new method --- adversarial advantage actor-critic (Adversarial A2C), which significantly improves the efficiency of dialogue policy learning in task-completion dialogue systems. Inspired by generative adversarial networks (GAN), we train a discriminator to differentiate responses/actions generated by dialogue agents from responses/actions by experts.
- Categories:
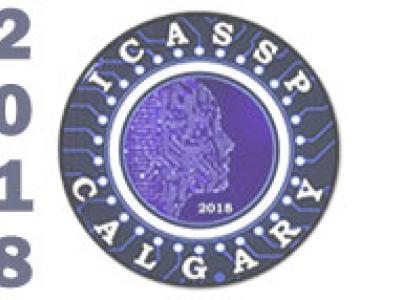
- Read more about Attention-based Dialog State Tracking for Conversational Interview Coaching
- Log in to post comments
This study proposes an approach to dialog state tracking (DST) in a conversational interview coaching system. For the interview coaching task, the semantic slots, used mostly in traditional dialog systems, are difficult to define manually. This study adopts the topic profile of the response from the interviewee as the dialog state representation. In addition, as the response generally consists of several sentences, the summary vector obtained from a long short-term memory neural network (LSTM) is likely to contain noisy information from many irrelevant sentences.
- Categories:
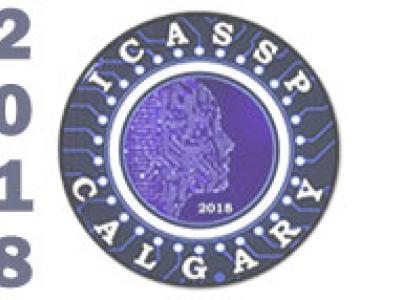
- Read more about Attention-based Dialog State Tracking for Conversational Interview Coaching
- Log in to post comments
This study proposes an approach to dialog state tracking (DST) in a conversational interview coaching system. For the interview coaching task, the semantic slots, used mostly in traditional dialog systems, are difficult to define manually. This study adopts the topic profile of the response from the interviewee as the dialog state representation. In addition, as the response generally consists of several sentences, the summary vector obtained from a long short-term memory neural network (LSTM) is likely to contain noisy information from many irrelevant sentences.
- Categories: