Documents
Presentation Slides
Presentation of Diffusion-based speech enhancement with a weighted generative-supervised learning loss
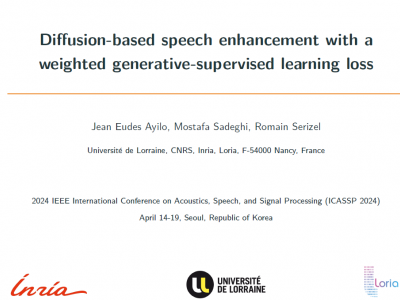
- Citation Author(s):
- Submitted by:
- Jean-Eudes AYILO
- Last updated:
- 15 April 2024 - 11:09pm
- Document Type:
- Presentation Slides
- Event:
- Presenters:
- Jean-Eudes AYILO
- Categories:
- Log in to post comments
Diffusion-based generative models have recently gained attention in speech enhancement (SE), providing an alternative to conventional supervised methods. These models transform clean speech training samples into Gaussian noise, usually centered on noisy speech, and subsequently learn a parameterized
model to reverse this process, conditionally on noisy speech. Unlike supervised methods, generative-based SE approaches often rely solely on an unsupervised loss, which may result in less efficient incorporation of conditioned noisy speech. To address this issue, we propose augmenting the original diffusion training objective with an ℓ2 loss, measuring the discrepancy between ground-truth clean speech and its estimation at each diffusion time-step. Experimental results demonstrate the effectiveness of our proposed methodology.
Comments
Diffusion-based speech
Diffusion-based speech enhancement with a weighted generative-supervised learning loss