Documents
Presentation Slides
Probabilistic Fine-grained Urban Flow Inference with Normalizing Flows
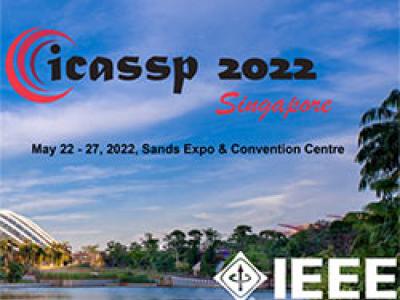
- Citation Author(s):
- Submitted by:
- Haoyang Yu
- Last updated:
- 5 May 2022 - 9:55am
- Document Type:
- Presentation Slides
- Document Year:
- 2022
- Event:
- Presenters:
- Haoyang Yu
- Paper Code:
- MLSP-20.2
- Categories:
- Log in to post comments
Fine-grained urban flow inference (FUFI) aims at enhancing the resolution of traffic flow, which plays an important role in intelligent traffic management. Existing FUFI methods are mainly based on techniques from image super-resolution (SR) models, which cannot fully capture the influence of external factors and face the ill-posed problem in SR tasks. In this paper, we propose UFI-Flow – Urban Flow Inference via normalizing Flow, a novel model for addressing the FUFI problem in a principled manner by using a single probabilistic loss. UFI-Flow therefore directly accounts for the ill-posed nature of the problem and learns spatial correlations between urban flow maps. In addition, an augmented distribution fusion mechanism is further proposed to reinforce the influence of external factors in the joint distribution inference. We conduct comprehensive experiments on real-world datasets to show the superiority of the proposed model compared to the state-of-the-art baseline approaches.
ICASSP_slides.pptx

ICASSP_5513_poster.pdf
