Documents
Presentation Slides
PROMPTING LARGE LANGUAGE MODELS WITH FINE-GRAINED VISUAL RELATIONS FROM SCENE GRAPH FOR VISUAL QUESTION ANSWERING
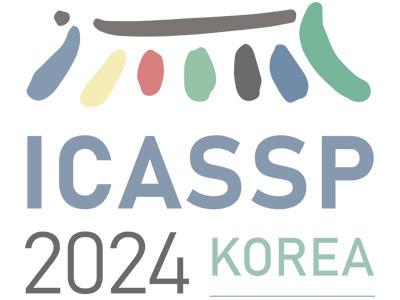
- DOI:
- 10.60864/s5mx-qk95
- Citation Author(s):
- Submitted by:
- P Liu
- Last updated:
- 6 June 2024 - 10:23am
- Document Type:
- Presentation Slides
- Event:
- Categories:
- Log in to post comments
Visual Question Answering (VQA) is a task that requires models to comprehend both questions and images. An increasing number of works are leveraging the strong reasoning capabilities of Large Language Models (LLMs) to address VQA. These methods typically utilize image captions as visual text description to aid LLMs in comprehending images. However, these captions often overlooking the relations of fine-grained objects, which will limit the reasoning capability of LLMs. In this paper, we present PFVR, a modular framework that Prompts LLMs with Fine-grained Visual Relationships for VQA. PFVR primarily consists of an answer-guided generation module (AGG) and a questionguided filtering module (QGF). The two modules can combine to extract the fine-grained visual relations from scene graph, which will finally serve as crucial context for LLMs to comprehend the image. Extensive experiments conducted on the popular VQA dataset, GQA, confirm PFVR achieves state-of-the-art results compared to other strong VQA competitors, demonstrating its exceptional effectiveness.