Documents
Presentation Slides
Protect Your Deep Neural Networks from Piracy
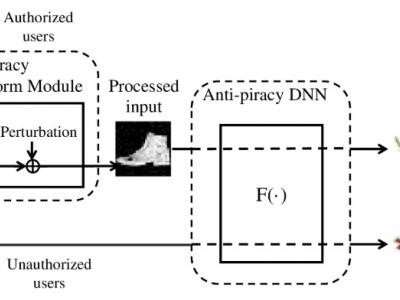
- Citation Author(s):
- Submitted by:
- Mingliang Chen
- Last updated:
- 5 February 2019 - 11:23am
- Document Type:
- Presentation Slides
- Document Year:
- 2018
- Paper Code:
- WIFS2018-96
- Categories:
- Log in to post comments
Building an effective DNN model requires massive human-labeled training data, powerful computing hardware and researchers' skills and efforts. Successful DNN models are becoming important intellectual properties for the model owners and should be protected from unauthorized access and piracy. This paper proposes a novel framework to provide access control to the trained deep neural networks so that only authorized users can utilize them properly. The proposed framework is capable of keeping the DNNs functional to authorized access while dysfunctional to unauthorized access or illicit use. The proposed framework is evaluated on the MNIST, Fashion, and CIFAR10 datasets to demonstrate its effectiveness to protect the trained DNNs from unauthorized access. The security of the proposed framework is examined against the potential attacks from unauthorized users. The experimental results show that the trained DNN models under the proposed framework maintain high accuracy to authorized access while having a low accuracy to unauthorized users, and they are resistant to several types of attacks.
Download/view the paper on IEEEXplore:
https://ieeexplore.ieee.org/document/8630791