Documents
Poster
PRUNING SIFT & SURF FOR EFFICIENT CLUSTERING OF NEAR-DUPLICATE IMAGES
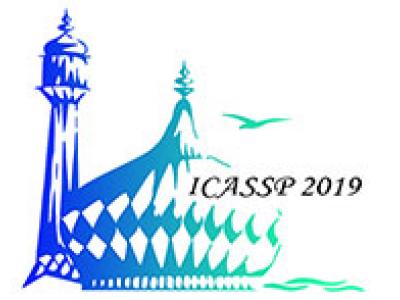
- Citation Author(s):
- Submitted by:
- Tushar Shinde
- Last updated:
- 9 May 2019 - 12:11am
- Document Type:
- Poster
- Document Year:
- 2019
- Event:
- Paper Code:
- 4574
- Categories:
- Log in to post comments
Clustering and categorization of similar images using SIFT and SURF require a high computational cost. In this paper, a simple approach to reduce the cardinality of keypoint set and prune the dimension of SIFT and SURF feature descriptors for efficient image clustering is proposed. For this purpose, sparsely spaced (uniformly distributed) important keypoints are chosen. In addition, multiple reduced dimensional variants of SIFT and SURF descriptors are presented. Moreover, clustering time complexity is also improved by proposed contextual bag-of-features approach for partitioned keypoint set. The F-measure statistic is used to evaluate clustering performance on a California-ND dataset containing near-duplicate images. Clustering accuracy of the proposed pruned SIFT and SURF is found to be at par with traditional SIFT and SURF with a significant reduction in computational cost.