Documents
Presentation Slides
QUANTIZED TENSOR ROBUST PRINCIPAL COMPONENT ANALYSIS
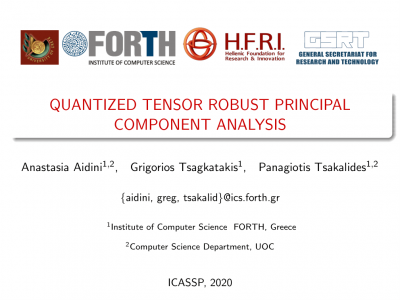
- Citation Author(s):
- Submitted by:
- Anastasia Aidini
- Last updated:
- 14 May 2020 - 7:54am
- Document Type:
- Presentation Slides
- Document Year:
- 2020
- Event:
- Categories:
- Log in to post comments
High-dimensional data structures, known as tensors, are fundamental in many applications, including multispectral imaging and color video processing. Compression of such huge amount of multidimensional data collected over time is of paramount importance, necessitating the process of quantization of measurements into discrete values. Furthermore, noise and issues related to the acquisition and transmission of signals frequently lead to unobserved, lost or corrupted measurements. In this paper, we introduce a tensor robust principal component analysis algorithm in order to recover a tensor with real-valued entries from a partly observed set of quantized and sparsely corrupted entries. We formulate the problem as a constrained maximum likelihood estimation of the sum of a low-rank tensor and a sparse tensor, through matricizations in each mode, in combination with a quantization and statistical measurement model. Experimental results on satellite derived land surface time-series demonstrate that directly operating with the quantized measurements, rather than treating them as real values, results in a low recovery error, while the proposed method is also capable of detecting temperature anomalies (e.g., forest fires).