Documents
Poster
QVRF: A QUANTIZATION-ERROR-AWARE VARIABLE RATE FRAMEWORK FOR LEARNED IMAGE COMPRESSION
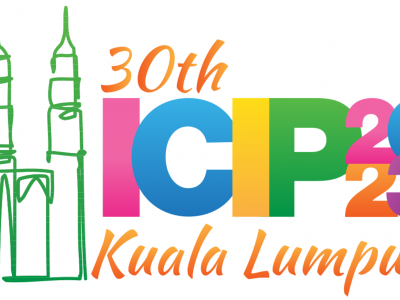
- DOI:
- 10.60864/6n45-1w49
- Citation Author(s):
- Submitted by:
- Xin Jin
- Last updated:
- 17 November 2023 - 12:05pm
- Document Type:
- Poster
- Document Year:
- 2023
- Event:
- Presenters:
- Kedeng Tong
- Categories:
- Log in to post comments
Learned image compression has exhibited promising compression performance, but variable bitrates over a wide range remain a challenge. State-of-the-art variable rate methods compromise the loss of model performance and require numerous additional parameters. In this paper, we present a Quantization-error-aware Variable Rate Framework (QVRF) that utilizes a univariate quantization regulator a to achieve wide-range variable rates within a single model. Specifically, QVRF defines a quantization regulator vector coupled with predefined Lagrange multipliers to control quantization error of all latent representation for discrete variable rates. Additionally, a reparameterization method makes QVRF compatible with round quantizer and integer entropy coding. Exhaustive experiments demonstrate that existing fixed-rate VAE-based methods equipped with QVRF can achieve wide-range continuous variable rates within a single model without significant performance degradation. Furthermore, QVRF outperforms contemporary variable-rate methods in rate-distortion performance with minimal additional parameters. The code is available at https://github.com/bytedance/QRAF.