Documents
Presentation Slides
RADAR+RGB Fusion for Robust Object Detection in Autonomous Vehicles
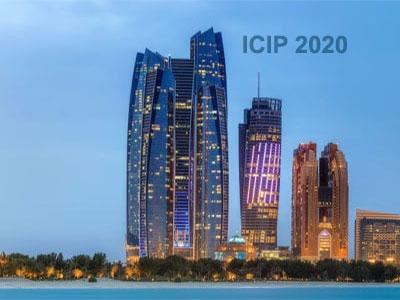
- Citation Author(s):
- Submitted by:
- Ritu Yadav
- Last updated:
- 2 November 2020 - 1:30pm
- Document Type:
- Presentation Slides
- Document Year:
- 2020
- Event:
- Presenters:
- Ritu Yadav
- Paper Code:
- 1953
- Categories:
- Log in to post comments
This paper presents two variations of architecture referred to as RANet and BIRANet. The proposed architecture aims to use radar signal data along with RGB camera images to form a robust detection network that works efficiently, even in variable lighting and weather conditions such as rain, dust, fog, and others. First, radar information is fused in the feature extractor network. Second, radar points are used to generate guided anchors. Third, a method is proposed to improve region proposal network targets. BIRANet yields 72.3/75.3% average AP/AR on the NuScenes dataset, which is better than the performance of our base network Faster-RCNN with Feature pyramid network(FFPN). RANet gives 69/71.9% average AP/AR on the same dataset, which is reasonably acceptable performance. Also, both BIRANet and RANet are evaluated to be robust towards the noise.