Documents
Poster
Rate-optimal Meta Learning of Classification Error
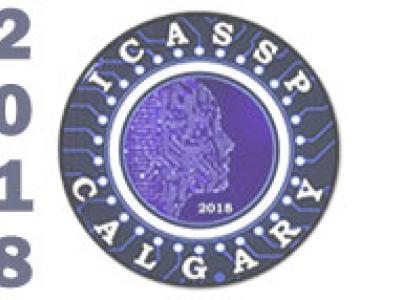
- Citation Author(s):
- Submitted by:
- Morteza Noshad
- Last updated:
- 16 April 2018 - 12:28am
- Document Type:
- Poster
- Document Year:
- 2018
- Event:
- Presenters:
- Alfred O. Hero
- Paper Code:
- MLSP-P2.7
- Categories:
- Log in to post comments
Meta learning of optimal classifier error rates allows an experimenter to empirically estimate the intrinsic ability of any estimator to discriminate between two populations, circumventing the difficult problem of estimating the optimal Bayes classifier. To this end we propose a weighted nearest neighbor (WNN) graph estimator for a tight bound on the Bayes classification error; the Henze-Penrose (HP) divergence. Similar to recently proposed HP estimators [berisha2016], the proposed estimator is non-parametric and does not require density estimation. However, unlike previous approaches the proposed estimator is rate-optimal, i.e., its mean squared estimation error (MSEE) decays to zero at the fastest possible rate of O(1/M+1/N) where M,N are the sample sizes of the respective populations. We illustrate the proposed WNN meta estimator for several simulated and real data sets.