Documents
Demo
Real-time perceptually motivated neural network for echo control and noise reduction
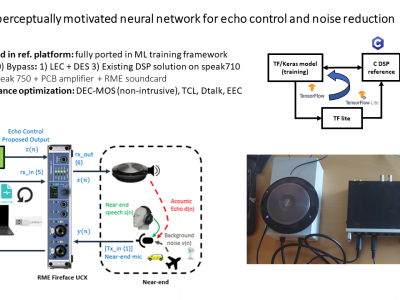
- DOI:
- 10.60864/grbq-1n60
- Citation Author(s):
- Submitted by:
- Pejman Mowlaee
- Last updated:
- 17 November 2023 - 12:07pm
- Document Type:
- Demo
- Document Year:
- 2023
- Event:
- Presenters:
- Pejman Mowlaee
- Paper Code:
- ST-L3.03
- Categories:
- Log in to post comments
Echo and background noise are the major obstacles in today’s user sound experience for devices like a speakerphone or video bar. We propose real-time perceptually motivated neural network-based echo control and noise reduction. The demonstrated method relies on a linear acoustic echo canceller (LAEC) combined with a neural network as a post-filter which incorporates perceptual mapping in both feature representation and loss function. The proposed method relies on mic and far-end signals for the LAEC stage, while the LAEC output, mic and echo estimate are inputs to the post-filter. The proposed hybrid approach links between signal processing, psychoacoustics, and neural network so it fits best to the theme of this year ICASSP (Signal Processing in the AI Era).
To provide an interactive demo and a transparent peer-to-peer connection, we use Jitsi WebRTC allowing the participants to experience the echo-annoyance of their own voice and the possibility to evaluate the offered benefits during an online conversation. We demonstrate the single-talk near-end, single-talk far-end and double-talk condition. As challenging scenarios, we will also include echo path change, movements in the room, touching the device, all known as challenging scenarios for echo control. In our setup, we use a speakerphone at one side and headset at the other. We demonstrate our real-time live demo using a Jabra Speak device where we have access to the raw mic and loudspeaker signals while processing our method in C code on the edge.
The footprint, real-time factor, and memory of the method allow presentation in real-time on a standard CPU without any look-ahead. It achieves an on-par performance compared to SOTA neural AEC solutions offering an attractive trade-off between echo leak, double talk and near end speech quality. It is also capable of handling background noise and transient noise. The proposed demo allows listening to three modes: no processing, LAEC, LAEC + residual echo suppressor.