Documents
Presentation Slides
Reconstruction of FRI Signals Using Deep Neural Network Approaches
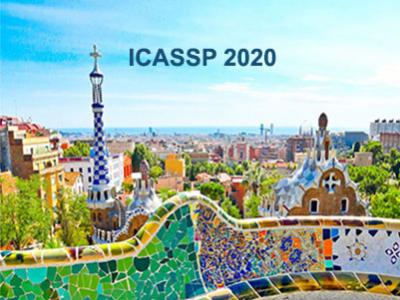
- Citation Author(s):
- Submitted by:
- Vincent C. H. Leung
- Last updated:
- 14 May 2020 - 7:28am
- Document Type:
- Presentation Slides
- Event:
- Paper Code:
- SPTM-L5.4
- Categories:
- Log in to post comments
Finite Rate of Innovation (FRI) theory considers sampling and reconstruction of classes of non-bandlimited continuous signals that have a small number of free parameters, such as a stream of Diracs. The task of reconstructing FRI signals from discrete samples is often transformed into a spectral estimation problem and solved using Prony's method and matrix pencil method which involve estimating signal subspaces. They achieve an optimal performance given by the Cramér-Rao bound yet break down at a certain peak signal-to-noise ratio (PSNR). This is probably due to the so-called subspace swap event. In this paper, we aim to alleviate the subspace swap problem and investigate alternative approaches including directly estimating FRI parameters using deep neural networks and utilising deep neural networks as denoisers to reduce the noise in the samples. Simulations show significant improvements on the breakdown PSNR over existing FRI methods, which still outperform learning-based approaches in medium to high PSNR regimes.