Documents
Presentation Slides
Reducing Image Compression Artifacts for Deep Neural Networks
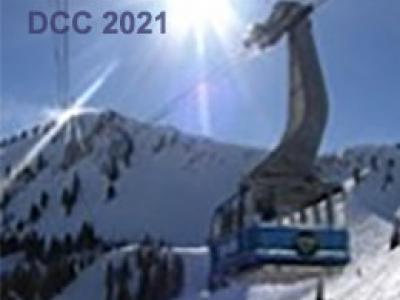
- Citation Author(s):
- Submitted by:
- Li Ma
- Last updated:
- 1 March 2021 - 10:11am
- Document Type:
- Presentation Slides
- Document Year:
- 2021
- Event:
- Presenters:
- Li Ma
- Paper Code:
- 108
- Categories:
- Log in to post comments
Existing compression artifacts reduction methods aim to restore images on pixel-level, which can improves human visual experience. However, in many applications, large-scale images are collected not for visual examination by human. Instead, they are used for many high-level vision tasks usually by Deep Neural Networks (DNN). One fundamental problem here is whether existing artifacts reduction methods can help DNNs improve the performance of the high-level tasks. In this paper, we find that these methods have limited performance improvements to high-level tasks, even bring negative effects. Therefore, inspired by the teacher-student network framework, we propose a compression artifacts reduction framework (ARF) for DNN. In addition, we generalize our method to the unsupervised setting (U-ARF) where the correspond original images are unavailable in training. Extensive experiments on image classification and person re-identification indicate the proposed methods can help DNNs improve performance on the highly compressed images significantly.