Documents
Poster
Regularized Gradient Descent Training of Steered Mixture of Experts for Sparse Image Representation
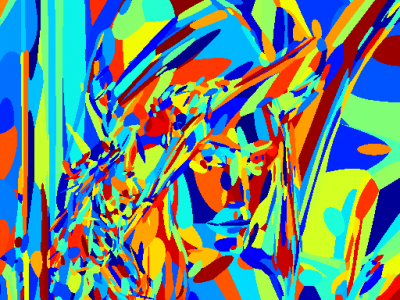
- Citation Author(s):
- Submitted by:
- Erik Bochinski
- Last updated:
- 5 October 2018 - 3:27am
- Document Type:
- Poster
- Document Year:
- 2018
- Event:
- Presenters:
- Erik Bochinski
- Paper Code:
- 2033
- Categories:
- Log in to post comments
The Steered Mixture-of-Experts (SMoE) framework targets a sparse space-continuous representation for images, videos, and light fields enabling processing tasks such as approximation, denoising, and coding.
The underlying stochastic processes are represented by a Gaussian Mixture Model, traditionally trained by the Expectation-Maximization (EM) algorithm.
We instead propose to use the MSE of the regressed imagery for a Gradient Descent optimization as primary training objective.
Further, we extend this approach with regularization terms to enforce desirable properties like the sparsity of the model or noise robustness of the training process.
Experimental evaluations show that our approach outperforms the state-of-the-art consistently by 1.5 dB to 6.1 dB PSNR for image representation.