Documents
Presentation Slides
Robust graph-filter identification with graph-denoising regularization
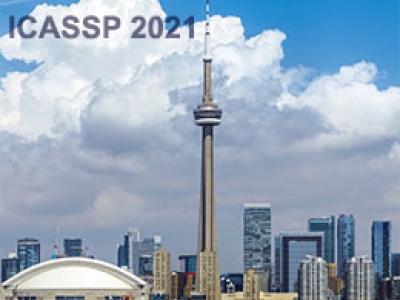
- Citation Author(s):
- Submitted by:
- Samuel Rey
- Last updated:
- 22 June 2021 - 3:52am
- Document Type:
- Presentation Slides
- Document Year:
- 2021
- Event:
- Presenters:
- Samuel Rey
- Paper Code:
- SPTM-12.6
- Categories:
- Keywords:
- Log in to post comments
When approaching graph signal processing tasks, graphs are usually assumed to be perfectly known. However, in many practical applications, the observed (inferred) network is prone to perturbations which, if ignored, will hinder performance. Tailored to those setups, this paper presents a robust formulation for the problem of graph-filter identification from input-output observations. Different from existing works, our approach consists in addressing the robust identification by formulating a joint graph denoising and graph-filter identification problem. Such a problem is formulated as a non-convex optimization, suitable relaxations are proposed, and graph-stationarity assumptions are incorporated to enhance performance. Finally, numerical experiments with synthetic and real-world graphs are used to assess the proposed schemes and compare them with existing (robust) alternatives.