Documents
Poster
Robust Recognition of Speech with Background Music in Acoustically Under-Resourced Scenarios
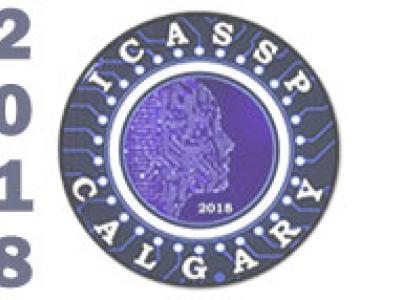
- Citation Author(s):
- Submitted by:
- Jiri Malek
- Last updated:
- 12 April 2018 - 11:32am
- Document Type:
- Poster
- Document Year:
- 2018
- Event:
- Presenters:
- Jiri Malek
- Paper Code:
- SP-P13.6
- Categories:
- Log in to post comments
This paper addresses the task of Automatic Speech Recognition
(ASR) with music in the background. We consider two different
situations: 1) scenarios with very small amount of labeled training
utterances (duration 1 hour) and 2) scenarios with large amount of
labeled training utterances (duration 132 hours). In these situations,
we aim to achieve robust recognition. To this end we investigate
the following techniques: a) multi-condition training of the acoustic
model, b) denoising autoencoders for feature enhancement and c)
joint training of both above mentioned techniques.
We demonstrate that the considered methods can be successfully
trained with the small amount of labeled acoustic data. We present
substantially improved performance compared to acoustic models
trained on clean speech. Further, we show a significant increase of
accuracy in the under-resourced scenario, when utilizing additional
amount of non-labeled data. Here, the non-labeled dataset is used to
improve the accuracy of the feature enhancement via autoencoders.
Subsequently, the autoencoders are jointly fine-tuned along with the
acoustic model using the small amount of labeled utterances.