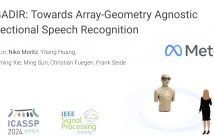
- Read more about AGADIR: Towards Array-Geometry Agnostic Directional Speech Recognition
- Log in to post comments
-
- Categories:
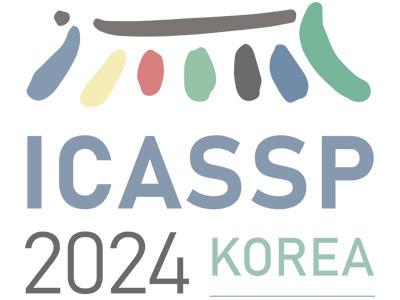
- Read more about Are Soft prompts good zero-shot learners for speech recognition?
- Log in to post comments
Large self-supervised pre-trained speech models require computationally expensive fine-tuning for downstream tasks. Soft prompt tuning offers a simple parameter-efficient alternative by utilizing minimal soft prompt guidance, enhancing portability while also maintaining competitive performance. However, not many people understand how and why this is so. In this study, we aim to deepen our understanding of this emerging method by investigating the role of soft prompts in automatic speech recognition (ASR).
- Categories:
- Read more about Unsupervised Accent Adaptation Through Masked Language Model Correction Of Discrete Self-Supervised Speech Units
- Log in to post comments
Self-supervised pre-trained speech models have strongly improved speech recognition, yet they are still sensitive to domain shifts and accented or atypical speech. Many of these models rely on quantisation or clustering to learn discrete acoustic units. We propose to correct the discovered discrete units for accented speech back to a standard pronunciation in an unsupervised manner. A masked language model is trained on discrete units from a standard accent and iteratively corrects an accented token sequence by masking unexpected cluster sequences and predicting their common variant.
- Categories:

- Read more about META REPRESENTATION LEARNING METHOD FOR ROBUST SPEAKER VERIFICATION IN UNSEEN DOMAINS
- Log in to post comments
This paper presents a meta representation learning method for robust speaker verification (SV) in unseen domains. It is known that the existing embedding learning based SV systems may suffer from domain mismatch issues. To address this, we propose an episodic training procedure to compensate domain mismatch conditions at runtime. Specifically, episodes are constructed with domain balanced episodic sampling from two different domains, and a new domain alignment (DA) module is added besides the feature extractor (FE) and classifier to existing network structures.
- Categories:
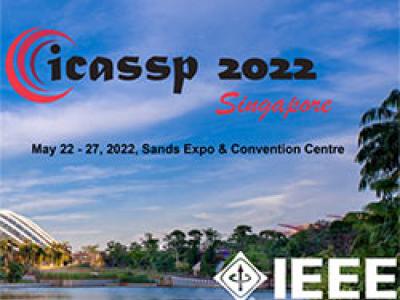
- Read more about Transducer-Based Streaming Deliberation For Cascaded Encoders
- Log in to post comments
Previous research on applying deliberation networks to automatic speech recognition has achieved excellent results. The attention decoder based deliberation model often works as a rescorer to improve first-pass recognition results, and requires the full first-pass hypothesis for second-pass deliberation. In this work, we propose a transducer-based streaming deliberation model. The joint network of a transducer decoder often receives inputs from the encoder and the prediction network. We propose to use attention to the first-pass text hypothesis as the third input to the joint network.
- Categories:
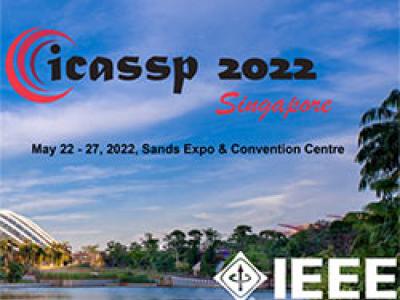
- Read more about Joint Speech Recognition and Audio Captioning
- Log in to post comments
Speech samples recorded in both indoor and outdoor environments are often contaminated with secondary audio sources. Most end-to-end monaural speech recognition systems either remove these background sounds using speech enhancement or train noise-robust models. For better model interpretability and holistic understanding, we aim to bring together the growing field of automated audio captioning (AAC) and the thoroughly studied automatic speech recognition (ASR). The goal of AAC is to generate natural language descriptions of contents in audio samples.
- Categories:
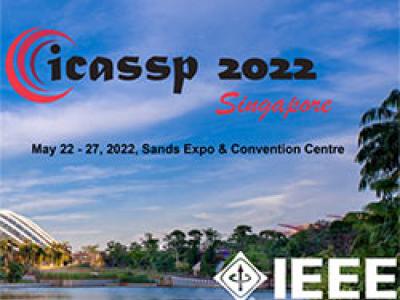
- Read more about Interactive Feature Fusion for End-to-End Noise-Robust Speech Recognition
- Log in to post comments
Speech enhancement (SE) aims to suppress the additive noise from noisy speech signals to improve the speech's perceptual quality and intelligibility. However, the over-suppression phenomenon in the enhanced speech might degrade the performance of downstream automatic speech recognition (ASR) task due to the missing latent information. To alleviate such problem, we propose an interactive feature fusion network (IFF-Net) for noise-robust speech recognition to learn complementary information from the enhanced feature and original noisy feature.
Hu_2783.pdf

- Categories:
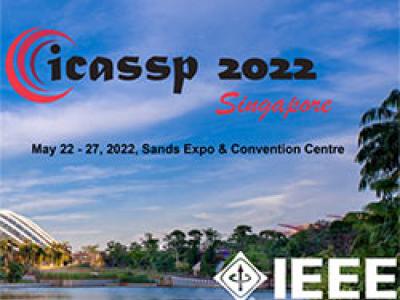
- Read more about WAV2VEC-SWITCH: CONTRASTIVE LEARNING FROM ORIGINAL-NOISY SPEECH PAIRS FOR ROBUST SPEECH RECOGNITION
- Log in to post comments
The goal of self-supervised learning (SSL) for automatic speech recognition (ASR) is to learn good speech representations from a large amount of unlabeled speech for the downstream ASR task. However, most SSL frameworks do not consider noise robustness which is crucial for real-world applications. In this paper we propose wav2vec-Switch, a method to encode noise robustness into contextualized representations of speech via contrastive learning. Specifically, we feed original-noisy speech pairs simultaneously into the wav2vec 2.0 network.
- Categories:
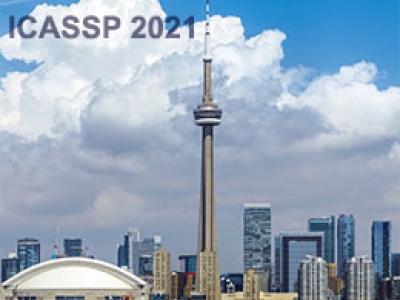
- Read more about Streaming Multi-Speaker ASR with RNN-T
- Log in to post comments
Recent research shows end-to-end ASR systems can recognize overlapped speech from multiple speakers. However, all published works have assumed no latency constraints during inference, which does not hold for most voice assistant inter- actions. This work focuses on multi-speaker speech recognition based on a recurrent neural network transducer (RNN-T) that has been shown to provide high recognition accuracy at a low latency online recognition regime.
- Categories:
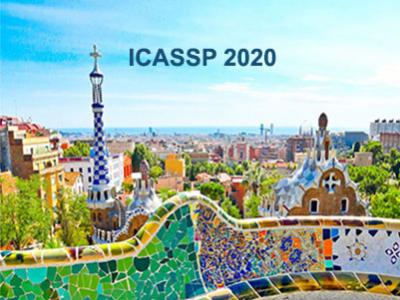
- Read more about Multi-scale Octave Convolutions for Robust Speech Recognition
- Log in to post comments
We propose a multi-scale octave convolution layer to learn robust speech representations efficiently. Octave convolutions were introduced by Chen et al [1] in the computer vision field to reduce the spatial redundancy of the feature maps by decomposing the output of a convolutional layer into feature maps at two different spatial resolutions, one octave apart. This approach improved the efficiency as well as the accuracy of the CNN models. The accuracy gain was attributed to the enlargement of the receptive field in the original input space.
- Categories: