Documents
Poster
Robust Sparse Learning Based on Kernel Non-second Order Minimization
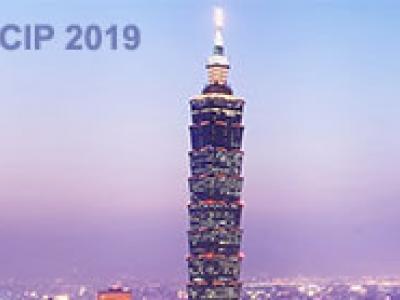
- Citation Author(s):
- Submitted by:
- Miaohua Zhang
- Last updated:
- 16 September 2019 - 4:45am
- Document Type:
- Poster
- Document Year:
- 2019
- Event:
- Presenters:
- Miaohua Zhang
- Paper Code:
- 2095
- Categories:
- Keywords:
- Log in to post comments
Partial occlusions in face images pose a great problem for most face recognition algorithms due to the fact that most of these algorithms mainly focus on solving a second order loss function, e.g., mean square error (MSE), which will magnify the effect from occlusion parts. In this paper, we proposed a kernel non-second order loss function for sparse representation (KNS-SR) to recognize or restore partially occluded facial images, which both take the advantages of the correntropy and the non-second order statistics measurement. The resulted framework is more accurate than the MSE-based ones in locating and eliminating outliers information. Experimental results from image reconstruction and recognition tasks on publicly available databases show that the proposed method achieves better performances compared with existing methods.
ICIP2019sparse.pdf
