Documents
Presentation Slides
Scale Selective Extended Local Binary Pattern For Texture Classification
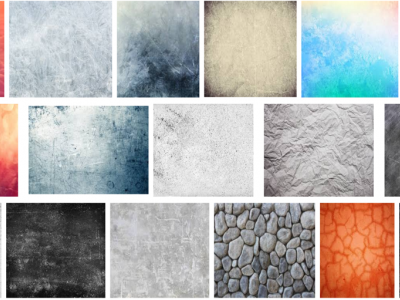
- Citation Author(s):
- Submitted by:
- Yuting Hu
- Last updated:
- 9 March 2017 - 3:18pm
- Document Type:
- Presentation Slides
- Document Year:
- 2017
- Event:
- Presenters:
- Yuting Hu
- Paper Code:
- IVMSP-L8.2
- Categories:
- Log in to post comments
In this paper, we propose a new texture descriptor, scale selective extended local binary pattern (SSELBP), to characterize texture images with scale variations. We first utilize multiscale extended local binary patterns (ELBP) with rotation invariant and uniform mappings to capture robust local micro and macro-features. Then, we build a scale space using Gaussian filters and calculate the histogram of multi-scale ELBPs for the image at each scale. Finally, we select the maximum values from the corresponding bins of multi-scale ELBP histograms at different scales as scale-invariant features. A comprehensive evaluation on public texture databases (KTH-TIPS and UMD) shows that the proposed SSELBP has high accuracy comparable to state-of-the-art texture descriptors on gray scale-,rotation-, and scale-invariant texture classification but uses only one-third of the feature dimension.