Documents
Poster
SELECTIVE MUTUAL LEARNING: AN EFFICIENT APPROACH FOR SINGLE CHANNEL SPEECH SEPARATION
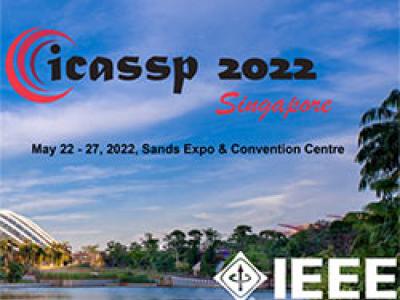
- Citation Author(s):
- Submitted by:
- Tan Ha
- Last updated:
- 30 May 2022 - 10:39am
- Document Type:
- Poster
- Event:
- Categories:
- Log in to post comments
Mutual learning, the related idea to knowledge distillation, is a group of untrained lightweight networks, which simultaneously learn and share knowledge to perform tasks together during training. In this paper, we propose a novel mutual learning approach, namely selective mutual learning. This is the simple yet effective approach to boost the performance of the networks for speech separation. There are two networks in the selective mutual learning method, they are like a pair of friends learning and sharing knowledge with each other. Especially, the high-confidence predictions are used to guide the remaining network while the low-confidence predictions are ignored. This helps to remove poor predictions of the two networks during sharing knowledge. The experimental results have shown that our proposed selective mutual learning method significantly improves the separation performance compared to existing training strategies including independently training, knowledge distillation, and mutual learning with the same network architecture.