Documents
Presentation Slides
Self-Supervised Face Image Restoration with a One-Shot Reference
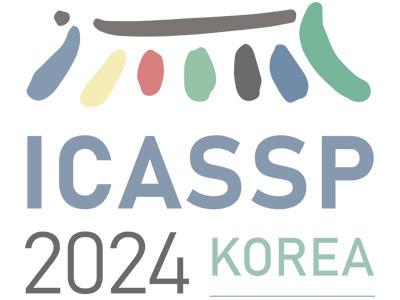
- DOI:
- 10.60864/xd5k-9x62
- Citation Author(s):
- Submitted by:
- Yanhui Guo
- Last updated:
- 6 June 2024 - 10:50am
- Document Type:
- Presentation Slides
- Document Year:
- 2024
- Event:
- Paper Code:
- IVMSP-L4.3
- Categories:
- Log in to post comments
For image restoration, methods leveraging priors from generative models have been proposed and demonstrated a promising capacity to robustly restore photorealistic and high-quality results. However, these methods are susceptible to semantic ambiguity, particularly with images that have obviously correct semantics, such as facial images. In this paper, we propose a semantic-aware latent space exploration method for image restoration (SAIR). By explicitly modelling semantic information from a given reference image, SAIR is able to reliably restore severely degraded images not only to high-resolution and highly realistic looks but also to correct semantics. Quantitative and qualitative experiments collectively demonstrate the superior performance of the proposed SAIR. Our code is available at https://github.com/Liamkuo/SAIR.