Documents
Presentation Slides
SELF-SUPERVISED MULTI-SCALE HIERARCHICAL REFINEMENT METHOD FOR JOINT LEARNING OF OPTICAL FLOW AND DEPTH
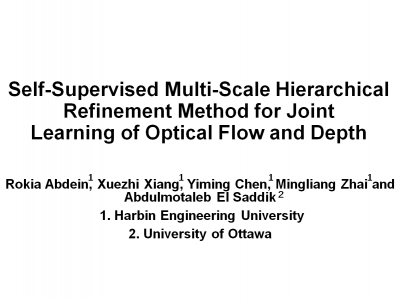
- DOI:
- 10.60864/pjg2-j270
- Citation Author(s):
- Submitted by:
- Yiming Chen
- Last updated:
- 6 June 2024 - 10:27am
- Document Type:
- Presentation Slides
- Document Year:
- 2024
- Event:
- Categories:
- Log in to post comments
Recurrently refining the optical flow based on a single highresolution feature demonstrates high performance. We exploit the strength of this strategy to build a novel architecture for the joint learning of optical flow and depth. Our proposed architecture is improved to work in the case of training on unlabeled data, which is extremely challenging. The loss is computed for the iterations carried out over a single high-resolution feature, where the reconstruction loss fails to optimize the accuracy particularity in occluded regions. Therefore, we propose to hierarchically refine the optical flow across multiple scales while feeding the rigid flow calculated from depth and camera pose to provide more refinement. We further propose a self-supervised patch-based similarity loss to be optimized with the reconstruction loss to improve accuracy in the occluded regions. Our proposed method demonstrates efficient performance on the KITTI 2015 dataset, with more improvement in the occluded regions.