Documents
Poster
Self-supervised Speaker Verification Employing a Novel Clustering Algorithm
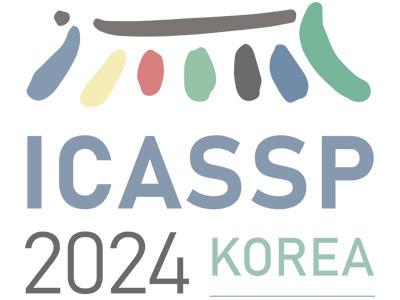
- DOI:
- 10.60864/ynwb-az32
- Citation Author(s):
- Submitted by:
- Abderrahim Fathan
- Last updated:
- 6 June 2024 - 10:28am
- Document Type:
- Poster
- Document Year:
- 2024
- Event:
- Presenters:
- Abderrahim Fathan
- Paper Code:
- 9492
- Categories:
- Log in to post comments
Clustering is an unsupervised learning technique, which leverages a large amount of unlabeled data to learn cluster-wise representations from speech. One of the most popular self-supervised techniques to train a speaker verification system is to predict the pseudo-labels using clustering algorithms and then train the speaker embedding network using the generated pseudo-labels in a discriminative manner. Therefore, pseudo-labels - driven self-supervised speaker verification systems' performance relies heavily on the accuracy of the adopted clustering algorithms. In this contribution, we propose a novel clustering technique that not only (i) combines predictions of augmented samples to provide a complementary supervisory signal for clustering and imposes symmetry within the augmentations but also (ii) enforces representation invariance via Self-Augmented Training (SAT) and maximizes the information-theoretic dependency between samples and their predicted pseudo-labels.
Experimental results on the VoxCeleb dataset show that the proposed clustering framework achieves better clustering performance in terms of a variety of clustering metrics. Proposed framework is also able to provide better self-supervised speaker verification performance than the state-of-the-art approaches trained on the same dataset.