Documents
Poster
SEMANTIC DISTILLATION AND STRUCTURAL ALIGNMENT NETWORK FOR FAKE NEWS DETECTION
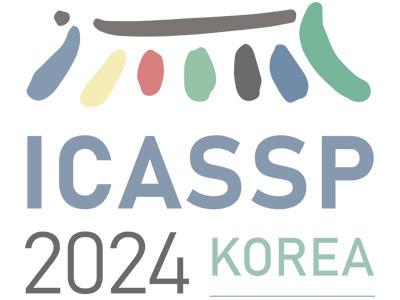
- DOI:
- 10.60864/g26k-hw53
- Citation Author(s):
- Submitted by:
- yue xiaofan
- Last updated:
- 7 April 2024 - 4:18am
- Document Type:
- Poster
- Document Year:
- 2024
- Event:
- Presenters:
- Yue Xiaofan
- Paper Code:
- MLSP-P29
- Categories:
- Log in to post comments
In recent years, the rapid proliferation of multi-modal fake news has posed potential harm across various sectors of society, making the detection of multi-modal fake news crucial. Most existing methods can not effectively reduce the redundant information and preserve both semantic and structural information. To address these problems, this paper proposes a semantic distillation and structural alignment (SDSA) network. We design an semantic distillation module for modality-specific features to preserve task-relevant semantic information and eliminate redundant information. Then, we propose a triple similarity alignment module to preserve structural information. Specifically, intra-modal similarity alignment mines intra-modal consistency by preserving the neighborhood structure within each modality, inter-modal similarity alignment explores cross-modality consistency by bringing the cross-modality feature neighborhood structures, and joint similarity alignment aims to preserve the structural information of fused features. Experiments conducted on two widely used fake news datasets demonstrate that the SDSA method outperforms state-of-the-art approaches.