Documents
Poster
Poster
SEMI-BLIND SPATIALLY-VARIANT DECONVOLUTION IN OPTICAL MICROSCOPY WITH LOCAL POINT SPREAD FUNCTION ESTIMATION BY USE OF CONVOLUTIONAL NEURAL NETWORK
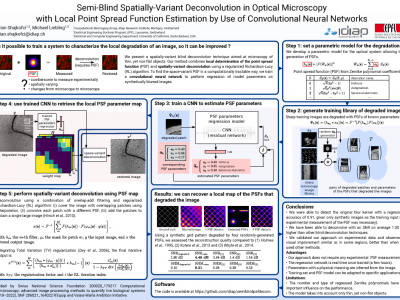
- Citation Author(s):
- Submitted by:
- Adrian Shajkofci
- Last updated:
- 6 October 2018 - 8:16pm
- Document Type:
- Poster
- Document Year:
- 2018
- Event:
- Presenters:
- Adrian Shajkofci
- Paper Code:
- WP.P9.1
- Categories:
- Log in to post comments
We present a semi-blind, spatially-variant deconvolution technique aimed at optical microscopy that combines a local estimation step of the point spread function (PSF) and deconvolution using a spatially variant, regularized Richardson-Lucy algorithm. To find the local PSF map in a computationally tractable way, we train a convolutional neural network to perform regression of an optical parametric model on synthetically blurred image patches. We deconvolved both synthetic and experimentally-acquired data, and achieved an improvement of image SNR of 1.00 dB on average, compared to other deconvolution algorithms.