Documents
Presentation Slides
SEQUENCE-TO-SUBSEQUENCE LEARNING WITH CONDITIONAL GAN FOR POWER DISAGGREGATION
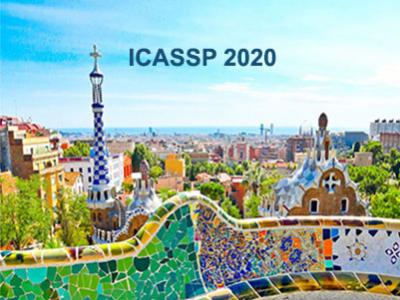
- Citation Author(s):
- Submitted by:
- yungang pan
- Last updated:
- 15 May 2020 - 5:10am
- Document Type:
- Presentation Slides
- Document Year:
- 2020
- Event:
- Presenters:
- Yungang Pan
- Paper Code:
- MLSP-L4.6
- Categories:
- Keywords:
- Log in to post comments
Non-intrusive load monitoring (a.k.a. power disaggregation) refers to identifying and extracting the consumption patterns of individual appliances from the mains which records the whole-house energy consumption. Recently, deep learning has been shown to be a promising method to solve this problem and many approaches based on it have been proposed. In this paper, we propose a sequence-to-subsequence learning method, which makes a trade-off between traditional sequence-to-sequence and sequence-to-point method, to balance the convergence difficulty in deep neural networks and the amount of computation in the inference period. We build our model based on conditional generative adversarial network that helps us avoid designing the loss function manually. In addition, we apply U-Net and Instance Normalization techniques to our model and demonstrate their effectiveness. Evaluations are performed on real-world data sets and we achieve the state-of-the-art performance.