Documents
Poster
SF-CNN: A Fast Compression Artifacts Removal via Spatial-to-Frequency Convolutional Neural Networks
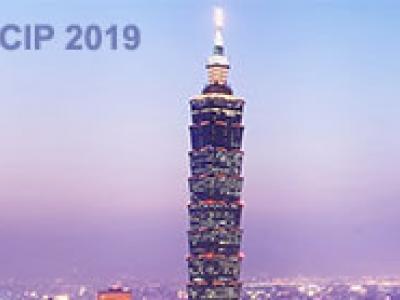
- Citation Author(s):
- Submitted by:
- Taeoh Kim
- Last updated:
- 18 September 2019 - 11:51pm
- Document Type:
- Poster
- Document Year:
- 2019
- Event:
- Presenters:
- Taeoh Kim
- Paper Code:
- WA.PH.7
- Categories:
- Log in to post comments
In this paper, we propose SF-CNN, a fast convolutional neural network structure for JPEG image compression artifacts removal. Recently, Convolutional Neural Network (CNN) based image restoration has shown great performance improvement. However, their heavy computational cost makes it difficult to apply other applications such as high-level vision tasks. Since heavy computation arises from maintaining spatial resolution of an input image, some works make a structure which is composed of spatial downsampling and
upsampling operations. SF-CNN takes Spatial input then predicts residual Frequency using downsampling operations only. Since every 8x8 pixel is grouped and spatially invariant in the JPEG DCT domain, It is possible to downsample the input by factor of 8 to reduce the computational cost. We show this simple structure is effective for compression artifacts removal. Our scalable baseline networks achieve comparative results compared to the reference networks in reduced computations.