Documents
Poster
SINGLE IMAGE DEPTH PREDICTION USING SUPER-COLUMN SUPER-PIXEL FEATURES
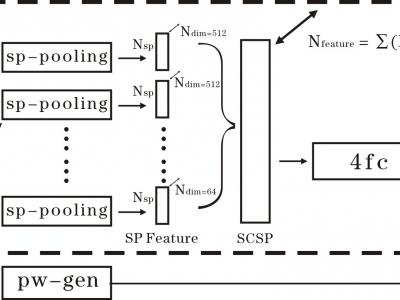
- Citation Author(s):
- Submitted by:
- Xufeng Guo
- Last updated:
- 15 September 2017 - 9:27pm
- Document Type:
- Poster
- Document Year:
- 2017
- Event:
- Presenters:
- Xufeng Guo
- Paper Code:
- 3442
- Categories:
- Log in to post comments
Depth prediction from a single monocular image is a challenging yet valuable task, as often a depth sensor is not available. The state-of-the-art approach \cite{Liu2016} combines a deep fully convolutional network (DFCN) with a conditional random field (CRF), allowing the CRF to correct and smooth the depth values estimated by the DFCN according to efficient contextual modeling.
However, using the output of the DFCN as unary input for CRF is limited by using only the last layer of the DFCN. The middle layers of the DFCN have been shown to carry useful information for other scene understanding tasks, which may help to improve the prediction quality.
This paper proposes a novel super-column super-pixel (SCSP) feature that is the combination of multiple layers of the DFCN after a super-pixel pooling process. The proposed approach based on the SCSP features reduces the root mean square (rms) error of the prediction by more than 16\% in NYUv2 dataset.
poster-surf.pdf
