Documents
Poster
SKIPPING MEMORY LSTM FOR LOW-LATENCY REAL-TIME CONTINUOUS SPEECH SEPARATION
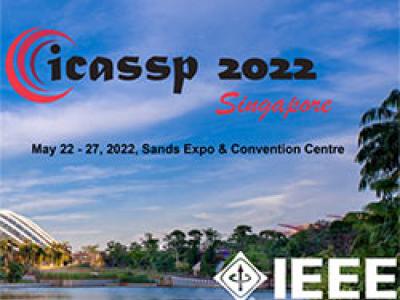
- Citation Author(s):
- Submitted by:
- Chenda Li
- Last updated:
- 11 May 2022 - 8:16am
- Document Type:
- Poster
- Event:
- Presenters:
- Chenda Li
- Paper Code:
- 4114
- Categories:
- Log in to post comments
Continuous speech separation for meeting pre-processing has recently become a focused research topic. Compared to the data in utterance-level speech separation, the meeting-style audio stream lasts longer, has an uncertain number of speakers. We adopt the time-domain speech separation method and the recently proposed Graph-PIT to build a super low-latency online speech separation model, which is very important for the real application. The low-latency time-domain encoder with a small stride leads to an extremely long feature sequence. We proposed a simple yet efficient model named Skipping Memory (SkiM) for the long sequence modeling. Experimental results show that SkiM achieves on par or even better separation performance than DPRNN. Meanwhile, the computational cost of SkiM is reduced by 75% compared to DPRNN. The strong long sequence modeling capability and low computational cost make SkiM a suitable model for online CSS applications. Our fastest real-time model gets 17.1 dB signal-to-distortion (SDR) improvement with less than 1-millisecond latency in the simulated meeting-style evaluation.
poster.pdf
