Documents
Presentation Slides
Slide for Interpretable Multimodal Out-of-context Detection with Soft Logic Regularization
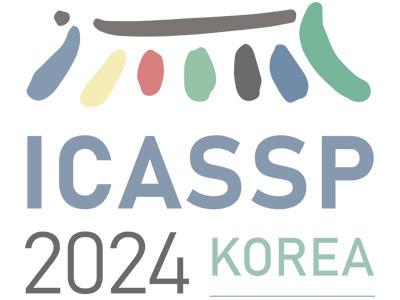
- DOI:
- 10.60864/8kts-cj26
- Citation Author(s):
- Submitted by:
- Ma Huanhuan
- Last updated:
- 6 June 2024 - 10:23am
- Document Type:
- Presentation Slides
- Document Year:
- 2024
- Event:
- Presenters:
- Huanhuan Ma
- Paper Code:
- IFS-L5.2
- Categories:
- Log in to post comments
The rapid spread of information through mobile devices and media has led to the widespread of false or deceptive news, causing significant concerns in society. Among different types of misinformation, image repurposing, also known as out-of-context misinformation, remains highly prevalent and effective. However, current approaches for detecting out-of-context misinformation often lack interpretability and offer limited explanations. In this study, we propose a logic regularization approach for out-of-context detection called LOGRAN (LOGic Regularization for out-of-context ANalysis). The primary objective of LOGRAN is to decompose the out-of-context detection at the phrase level. By employing latent variables for phrase-level predictions, the final prediction of the image-caption pair can be aggregated using logical rules. The latent variables also provide an explanation for how the final result is derived, making this fine-grained detection method inherently explanatory. We evaluate the performance of LOGRAN on the NewsCLIPpings dataset, showcasing competitive overall results. Visualized examples also reveal faithful phrase-level predictions of out-of-context images, accompanied by explanations. This highlights the effectiveness of our approach in addressing out-of-context detection and enhancing interpretability.