Documents
Poster
Solving Quadratic Equations via Amplitude-based Nonconvex Optimization
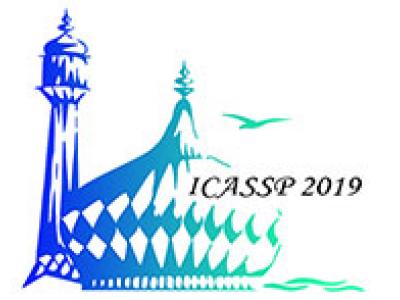
- Citation Author(s):
- Submitted by:
- Vincent Monardo
- Last updated:
- 10 May 2019 - 8:03am
- Document Type:
- Poster
- Document Year:
- 2019
- Event:
- Presenters:
- Vincent Monardo
- Paper Code:
- 1688
- Categories:
- Log in to post comments
In many signal processing tasks, one seeks to recover an r- column matrix object X ∈ Cn×r from a set of nonnegative quadratic measurements up to orthonormal transforms. Example applications include coherence retrieval in optical imaging and co- variance sketching for high-dimensional streaming data. To this end, efficient nonconvex optimization methods are quite appealing, due to their computational efficiency and scalability to large-scale problems. There is a recent surge of activities in designing noncon- vex methods for the special case r = 1, known as phase retrieval; however, very little work has studied the general rank-r setting. Motivated by the success of phase retrieval, in this paper we derive several algorithms which utilize the quadratic loss function based on amplitude measurements, including (stochastic) gradient descent and alternating minimization. Numerical experiments demonstrate their computational and statistical performances, highlighting the superior performance of stochastic gradient descent with appropri- ate mini-batch sizes.