Documents
Poster
SPARSE-CODED DYNAMIC MODE DECOMPOSITION ON GRAPH FOR PREDICTION OF RIVER WATER LEVEL DISTRIBUTION
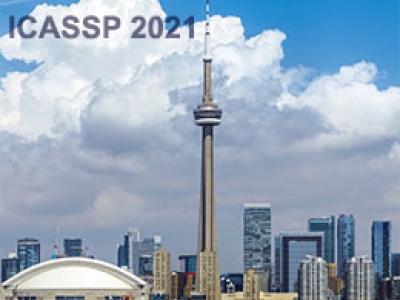
- Citation Author(s):
- Submitted by:
- Yusuke Arai
- Last updated:
- 30 July 2021 - 3:01am
- Document Type:
- Poster
- Document Year:
- 2021
- Event:
- Presenters:
- Yusuke Arai
- Paper Code:
- MLSP-17.5
- Categories:
- Log in to post comments
This work proposes a method for estimating dynamics on graph by using dynamic mode decomposition (DMD) and sparse approximation with graph filter banks (GFBs). The motivation of introducing DMD on graph is to predict multi-point river water levels for forecasting river flood and giving proper evacuation warnings. The proposed method represents a spatio-temporal variation of physical quantities on a graph as a time-evolution equation. Specifically, water level observation data available on the Internet is collected by web scraping. As well, the graph structure is defined based on numerical river information published by Ministry of Land, Infrastructure, Transport and Tourism (MILT) of Japan and the graph is used to construct GFBs for analyzing and synthesizing the water level data. GFBs work in combination with a sparse approximation algorithm for feature extraction of water level distribution. The features are exploited to derive the time-evolution equation through the extended DMD (EDMD) framework. The time-evolution equation is applied to predict river water level distribution. In order to verify the significance of the proposed method, the river water level prediction is conducted for real web-scraped data. The performance evaluation shows the superiority to the normal DMD approach.